Data: the Science, Analysis, and Engineering
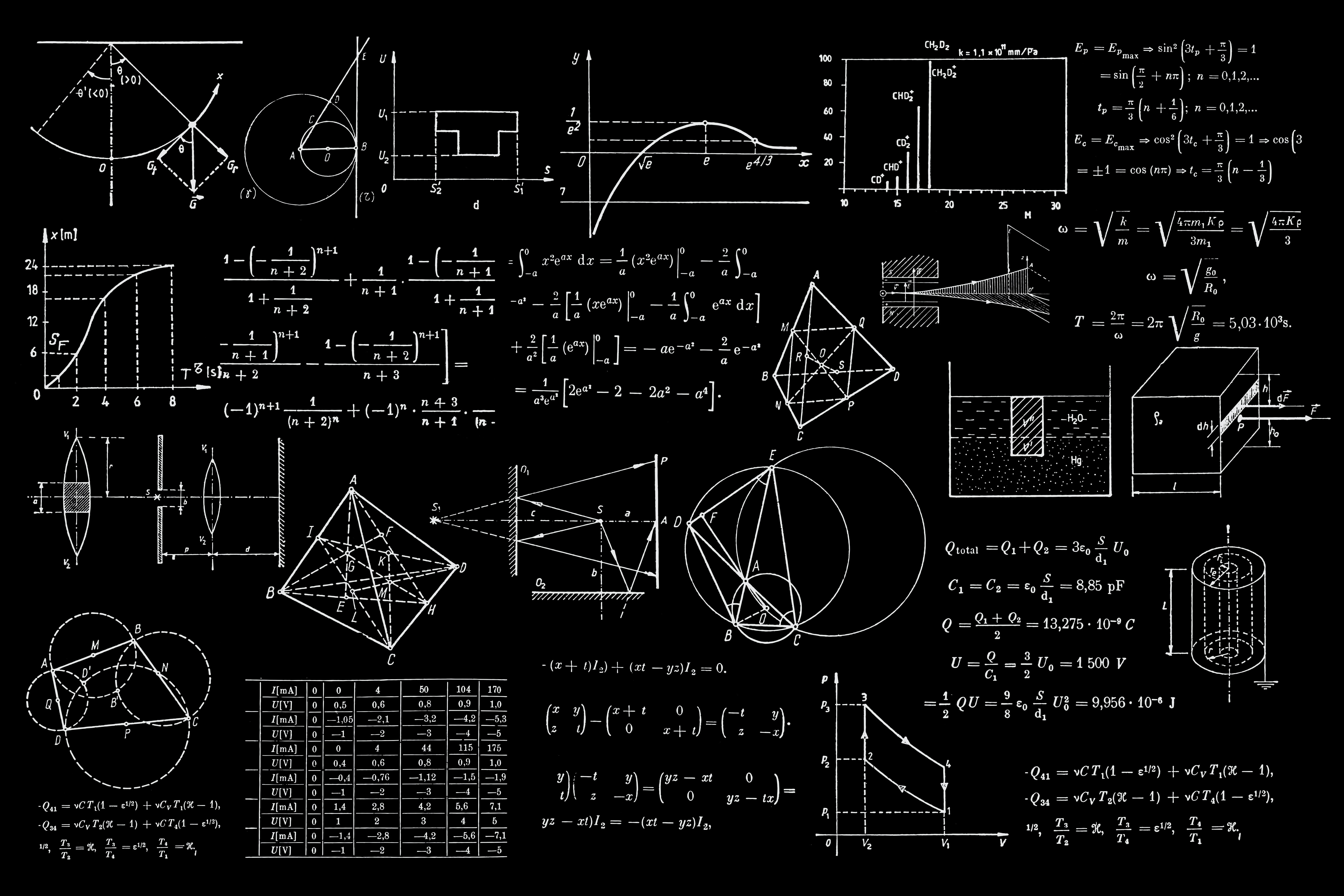
a.k.a. My foray into data
Some Context
Recently I decided to reconnect with my math and data loving roots and start to try and pivot in my career a little bit. This was a heck of a year to decide to do that, I had some major life changes including health woes, a cross country move, my mother passing away, and a startup initiative falling through. To say that this year is a lot is an extreme understatement.
In order to move forward I decided to optimize more on what I enjoy and try to align directly or adjacently with that.
What this means
Back when I went to college, and for all of my life before that, I wanted to be an astrophysicist. I wanted to study the origins of the universe and stellar evolution. All the cool stuff, and there is nothing really uncool about space, the final frontier. As I am writing this, it might be obvious that I am not an astrophysicist. I have been making my way through the tech industry though. The thoughts that I had in the beginning was that I could later find a job that was cross-disciplinary and possibly make use of various tech skills as well as the math and science needed for astronomy.
In short I don’t think I’ll be doing that specifically, but that doesn’t mean I can’t make choices that are more scientifically friendly. By that I mean be in pursuit of skills that will allow me to also answer the questions I have in my spare time. When I was younger that was all astronomy, of course, but as the climate crisis marches on and facsism is on the rise, I have questions about those. Fixing, mitigating, and avoiding if possible. All those are questions that mostly involve me needing to better understand maps and stats. This points to data work.
Learning what to learn
I’m going to be analyzing (:smirk:) how Data Science, Analysis, and Engineering titles differ practically speaking. I’m also going through searching and building a curriculum to use to acquire the knowledge for this space. I am looking at others already in this space to see what the comprehensive common core is. To put it another way, there are two major shortcomings I’ve noticed with a lot of materials when trying to learn. They either:
- Stop short / Fail to “Draw the Owl”
- Are too narrow in scope
For the first one, the big problem there is that finding the “101” level info that is beginner friendly is relatively easy. The more depth you want to learn, though, the harder it gets. This next meme probably looks familiar:
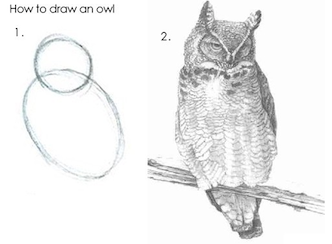
Draw the Owl meme
In trying to “build my curriculum”, I’m trying to do my best to get a list of the steps to actually Draw the Owl.
For the latter, the narrowness in scope isn’t only due to the Draw the Owl problem. The narrowness I am referring to here refers to the proliferation of materials that focus on “use X for this situation, use Y for this situation, use Z for this situation” without teaching the underlying concepts involved. This leads to an over-reliance on finding X, Y, and Z and all of their applicable situations rather than understanding the body of knowledge that’s being applied.
Although this hopefully makes sense, I’ve seen a lot of education and tutorials approaching from the “use this for X” perspective rather than “this is what X is”. A lot of pattern matching, frustration, and jokes about copy-pasta. I believe that no small amount of the “imposter syndrome” that plagues industries is from feeling this lack. (There are also separate discussions about institutional bias and prejudice that disproportionately impacts different demographics. That exceeds this specific discussion but it is necessary to call out.)
The most common issue I’ve encountered for narrowness is what I’m going call “capitalism scoped learning”. This is where you don’t learn statistics so much as learn business and only a piecemeal of stats of high relevance to business. If you were learning arithmetic in this style, you would less learn the operators and more learn “this is how you calculate sales tax and the total value of items on a receipt, itinerary, or bill of sale”. If you found yourself in a situation where you needed arithmetic outside of that scope, you might find you struggle more than if you learned arithmetic and then applied it to capitalism as well as any other needs. Similarly if you needed to do more complex arithmetic, if there were multiple tax rates, fees, and so on you would struggle to just factor them in because you wouldn’t necessarily actually understand arithmetic.
This is an obviously contrived example, but hopefully the point is still clear. Also, this isn’t to say that there is no benefit for learning via only extremely scoped applied cases as much as saying it cannot be the sole or primary source of learning. Since I am being specific, again I’m not calling out blog posts and fun side projects that are narrowly scoped, more calling out what I am noticing in “comprehensive learning tracks” that are not actually comprehensive.
Next Steps
Now that I have completed about 80% of my cross-country move I can actually give this more of my undivided attention. In the next post, or two depending on length, I’ll be documenting what I find out about:
- Figuring out how the industry distinguishes data scientist, data analyst, and data engineering roles
- Outlining topics and learning tracks in an attempt to make a curricula
I expect the latter will be a long, ongoing, work in progress that I’ll update multiple times across mutiple posts before I’m done.
Subsequent posts will be better organized as well, this is the first Gathering of Thoughts since settling in. Stay tuned!
Cover image by Dan Cristian Pădureț on Unsplash